徐茉莉 Galit Shmueli
Distinguished Professor, Institute of Service Science, National Tsing Hua University
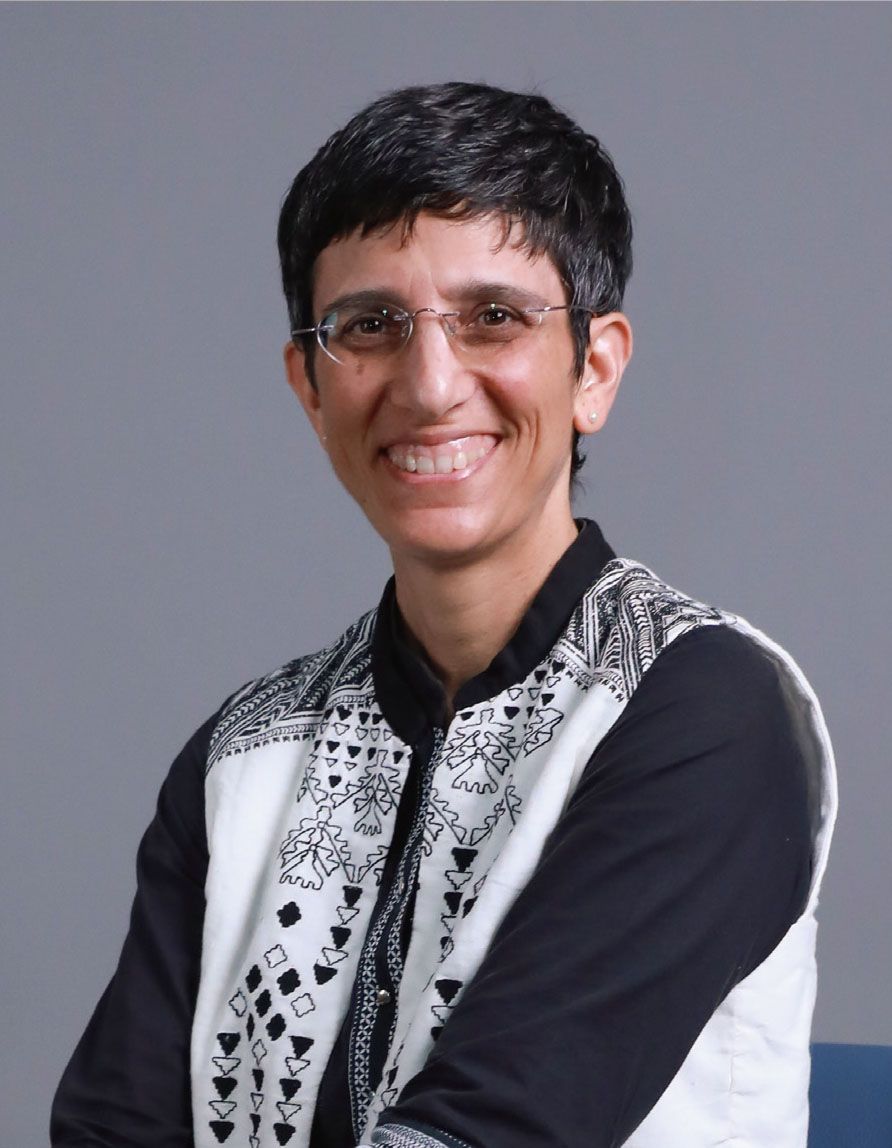
學歷
- Ph.D., Technion - Israel Institute of Technology, Israel (2000)
- M.Sc., Technion - Israel Institute of Technology, Israel (1997)
- B.A., University of Haifa, Israel (1994)
經歷
- Institute Director, Institute of Service Science, National Tsing Hua University (2020/8 ~ present)
- Editor-in-Chief, INFORMS Journal on Data Science (2020/5 ~ present)
- Distinguished Professor, Institute of Service Science, National Tsing Hua University (2014/8 ~ present)
針對行為大數據分析 開發統計和機器學習方法
Motivated by applications in information systems and healthcare, my research focuses on developing and evaluating statistical and machine learning approaches for analyzing behavioral big data. My research approach starts from identifying a modeling challenge that researchers or practitioners face, and then studying and developing appropriate methodologies.
My most notable research contribution is on causal explanation vs. prediction - understanding the differences between explanation and prediction, developing new predictive capabilities of explanatory models, and developing new causal tools from prediction algorithms. My paper “To Explain or To Predict?” (1900+ citations) examines fundamental and practical differences between using statistical and other empirical methods for prediction compared to causal explanation and to description. This paper has impacted multiple fields, including statistics, data mining, information systems, marketing, management, medicine, ecology, biology, environmental sciences, public policy, public health, and economics.
I have been expanding the explain/predict research by studying the possibilities of adapting machine learning algorithms intended for prediction for use in causal-explanatory tasks. And conversely, studying and extending the possibilities of explanatory models for prediction. I’ve been collaborating with colleagues in Taiwan, Germany, Israel, India, Australia and the USA. One direction is studying the predictive capabilities of causal-oriented Partial Least Squares Structural Equations Models (PLS), clarifying to the research community what type of prediction is available, how it can be carried out using PLS, and how PLS prediction can be used to advance scientific research.
A second direction is causal uses of Classification and Regression Trees, a popular machine learning algorithm used for prediction. We’ve adapted trees for causal analysis especially suitable for big data. We developed a computationally-feasible and theoretically-powerful approach that scales to big data, and applied it to several real cases including kidney allocation policies and new eGov services.
Finally, my recent research focuses on methodological, technical, and ethical issues that arise when using behavioral big data, large-scale behavioral randomized experiments, and behavior modification.
得獎感言
I am greatly honored to receive the Outstanding Research Award from the Ministry of Science and Technology. The award celebrates my research achievements obtained by joint efforts with my many sharpminded and good-hearted colleagues, collaborators, and students. The research award offers a wonderful milestone on the long and ongoing journey of discovery, co-creation, rigor, and creativity.
I am thankful also to those who create and support the positive and open research environment that I have been lucky to enjoy : my family, the Institute of Service Science at NTHU, the information systems research community, and the Ministry of Science and Technology.
個人勵志銘
When you come to a fork in the road, take it.─Yogi Berra
- 基礎研究超前部署
- 豐沛國家科研人才